Example 1: Resource
allocation for a rice breeding program using the GE model
Consider a rainfed lowland rice testing program in which the following
variances have been estimated in (t/ha)2
The table below shows the predicted effect of the number of trials and
replicates of testing on the standard deviation of a cultivar mean:
Number of sites |
Number of replicates/ site |
S.E. of cultivar mean
(t ha-1) |
LSD.05
for the difference between cultivar means (t ha-1) |
1 |
1 |
.87 |
2.61 |
|
2 |
.72 |
2.16 |
|
3 |
.67 |
2.01 |
|
4 |
.64 |
1.92 |
|
|
|
|
2 |
1 |
.61 |
1.83 |
|
2 |
.51 |
1.53 |
|
3 |
.47 |
1.41 |
|
4 |
.45 |
1.35 |
|
|
|
|
3 |
1 |
.50 |
1.50 |
|
2 |
.42 |
1.26 |
|
3 |
.39 |
1.17 |
|
4 |
.37 |
1.11 |
|
|
|
|
4 |
1 |
.43 |
1.29 |
|
2 |
.36 |
1.08 |
|
3 |
.34 |
1.02 |
|
4 |
.32 |
0.96 |
|
|
|
|
5 |
1 |
.39 |
1.08 |
|
2 |
.32 |
0.96 |
|
3 |
.30 |
0.90 |
|
4 |
.29 |
0.87 |
|
|
|
|
10 |
1 |
.27 |
0.81 |
|
2 |
.23 |
0.69 |
|
3 |
.21 |
0.63 |
|
4 |
.20 |
0.60 |
Several useful conclusions for planning further testing can
be drawn from this table:
The number of
trials is more important than the number of replicates per trial in determining
the precision with which cultivar means are estimated.
For this testing
system (and for many others), there is rarely much benefit from including
more than 3 replicates per trial.
When the number
of trials exceeds 3, there is unlikely to be much benefit to including
more than 2 replicates per trial.
Limitations of the genotype
x environment model in resource allocation planning
The model set out in Equation
has a serious limitation in its usefulness for the planning
of testing programs. In
this model, each trial is considered to be a separate environment. It
can be used to make decisions about how many replicates are warranted,
but it sheds no light on the number of years or locations at which trials
should be conducted. However, trials in a MET series are easily and naturally
categorized with respect to the year and location in which they were conducted.
If the environment term in Eq.
is partitioned into year and location effects, a more realistic
and informative model can be used for resource allocation exercises, permitting
informed decisions to be made about the number of locations, years, and
replicates required to achieve an adequate level of precision in cultivar
evaluation.
The genotype x site x year (GSY) model
A more realistic and complete model for the analysis of cultivar
trials than Eq.
recognizes years and sites as random factors used in sampling the TPE.
The resulting model is:

This model differs from the previous Eq. in that the E term
has been partitioned into site (S) and year (Y) effects and their interaction
(YS). Similarly,
the GE term has been partitioned into GY, GS, and GY components.
The variance of a cultivar mean is now expressed as:

Inspection of this last equation indicates that, if σ2GS
is large,
the variance of a cultivar mean can only be minimized if testing is conducted
at several sites.
Similarly, if σ2GY is large, testing
over several years will be required to achieve adequate precision in the
estimation of cultivar means. However,
if σ2GYS
is the largest component of GEI, then it may be possible to minimize
by either increasing the number of sites or the number of
years of testing.
Increasing the number of sites is expensive, but will produce
the desired information quickly.
Increasing the number of years of testing is less expensive
but will delay cultivar release.
Variance components for the GLY model can also be estimated
from the fully balanced ANOVA of a set of trials repeated over locations
and years:
Table 3. Expected
mean squares (EMS) for the ANOVA of the genotype x location x year (GLY)
model assuming all factors random.
Source |
Mean square |
EMS |
Years (Y) |
|
|
Sites (S) |
|
|
Y x S |
|
|
Replicates within Y x S |
|
|
Genotypes (G) |
MSG
|
σ2e + rσ2GYS + rsσ2GY+
ryσ2GS+ rysσ2G |
G x Y |
MSGS
|
σ2e + rσ2GYS + ryσ2GS |
G x S |
MSGY
|
σ2e + rσ2GYS + rsσ2GY |
G x Y x S |
MSGYS |
σ2e + rσ2GYS |
Plot residuals |
MSe
|
σ2e |
As for the GE model the variance components for the GLY model
can be estimated as functions of the mean squares estimated from the ANOVA:
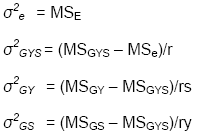